“R-Classify: Extracting Research Papers’ Relevant Concepts from a Controlled Vocabulary” is a software paper accepted at Software Impacts. Tanay Aggarwal, Angelo Antonio Salatino, Francesco Osborne, Enrico Motta Knowledge Media Institute, The Open University, Milton Keynes (UK) Abstract In the past few decades, we saw a proliferation of scientific articles available online. This data-rich environment offers several opportunities […]
Tag: semantic web

Leveraging Knowledge Graph Technologies to Assess Journals and Conferences at Springer Nature
“Leveraging Knowledge Graph Technologies to Assess Journals and Conferences at Springer Nature” is an In-Use paper presented at the 21st International Semantic Web Conference (ISWC 2022). Simone Angioni1, Angelo Antonio Salatino2, Francesco Osborne2,3,AliaksandrBirukou4, Diego Reforgiato Recupero1, Enrico Motta2 1 Department of Mathematics and Computer Science, University of Cagliari (Italy) 2 Knowledge Media Institute, The Open […]
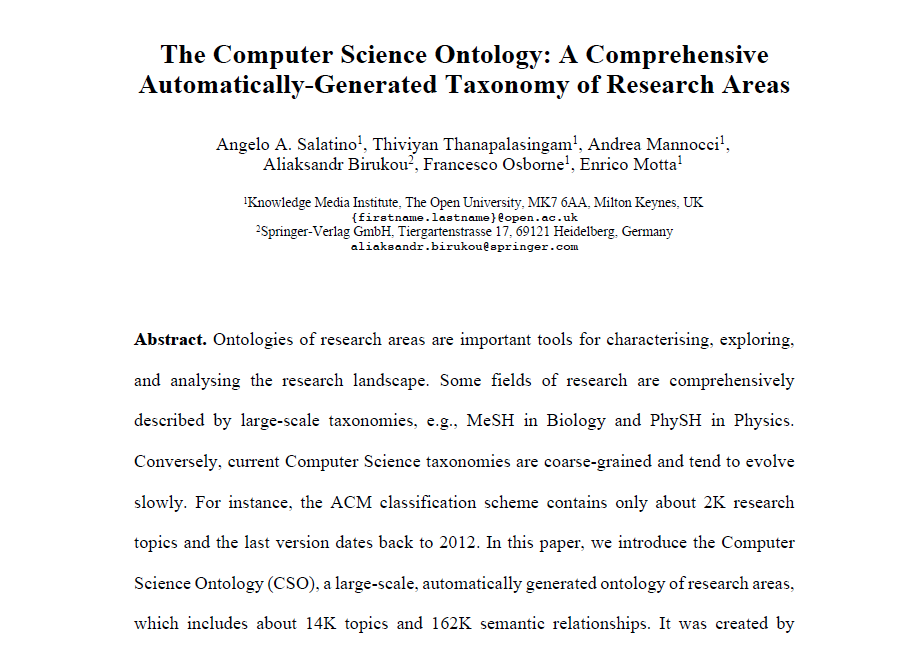
The Computer Science Ontology: A Comprehensive Automatically-Generated Taxonomy of Research Areas
Ontologies of research areas are important tools for characterising, exploring, and analysing the research landscape. Some fields of research are comprehensively described by large-scale taxonomies, e.g., MeSH in Biology and PhySH in Physics. Conversely, current Computer Science taxonomies are coarse-grained and tend to evolve slowly. For instance, the ACM classification scheme contains only about 2K research topics and the last version dates back to 2012. In this paper, we introduce the Computer Science Ontology (CSO), a large-scale, automatically generated ontology of research areas, which includes about 14K topics and 162K semantic relationships. It was created by applying the Klink-2 algorithm on a very large dataset of 16M scientific articles. CSO presents two main advantages over the alternatives: i) it includes a very large number of topics that do not appear in other classifications, and ii) it can be updated automatically by running Klink-2 on recent corpora of publications. CSO powers several tools adopted by the editorial team at Springer Nature and has been used to enable a variety of solutions, such as classifying research publications, detecting research communities, and predicting research trends. To facilitate the uptake of CSO, we have also released the CSO Classifier, a tool for automatically classifying research papers, and the CSO Portal, a web application that enables users to download, explore, and provide granular feedback on CSO. Users can use the portal to navigate and visualise sections of the ontology, rate topics and relationships, and suggest missing ones. The portal will support the publication of and access to regular new releases of CSO, with the aim of providing a comprehensive resource to the various research communities engaged with scholarly data.
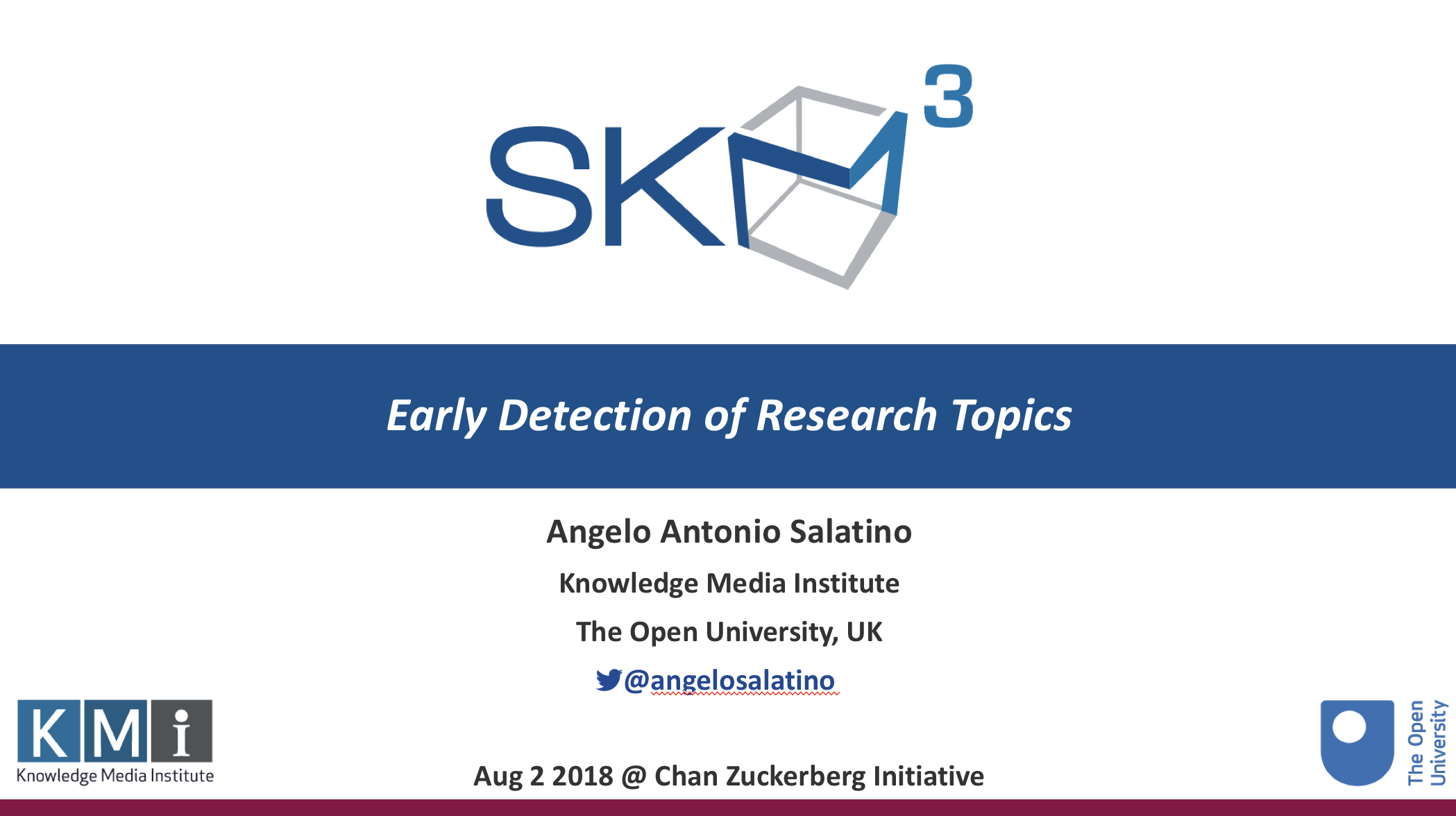
Invited Talk – Early detection of Research Topics
On 2nd of August 2018, I have been invited by Boris Veytsman, Principal Research Scientist at Chan Zuckerberg Initiative (formerly Meta), to give a talk about my PhD work. Differently from my previous talk to the ORNL group, I had the opportunity to describe my doctoral work more comprehensively. More specifically, I initially showed what is available […]
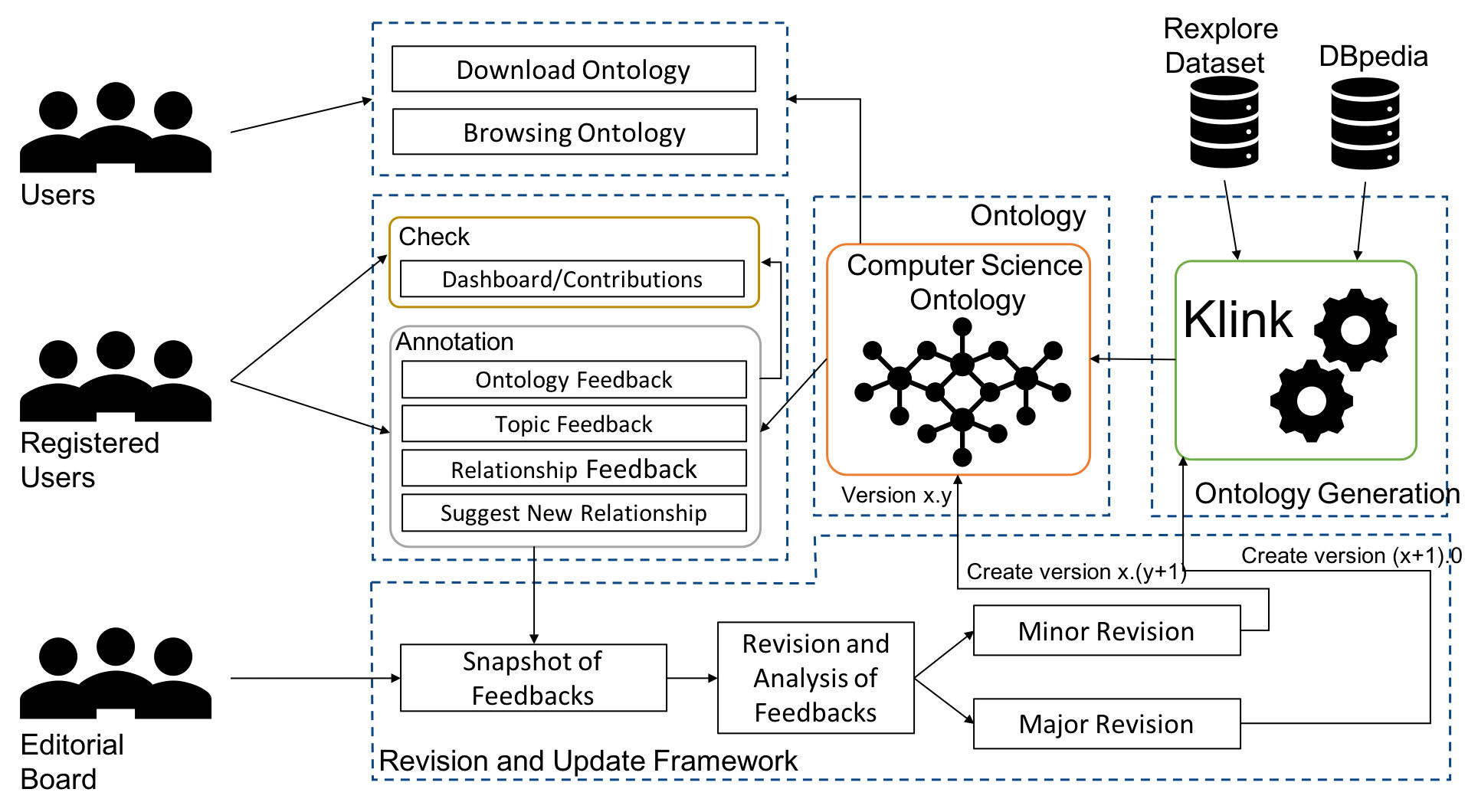
The Computer Science Ontology: A Large-Scale Taxonomy of Research Areas
Ontologies of research areas are important tools for characterising, exploring, and analysing the research landscape. Some fields of research are comprehensively described by large-scale taxonomies, e.g., MeSH in Biology and PhySH in Physics. Conversely, current Computer Science taxonomies are coarse-grained and tend to evolve slowly. For instance, the ACM classification scheme contains only about 2K research topics and the last version dates back to 2012. In this paper, we introduce the Computer Science Ontology (CSO), a large-scale, automatically generated ontology of research areas, which includes about 26K topics and 226K semantic relationships. It was created by applying the Klink-2 algorithm on a very large dataset of 16M scientific articles.
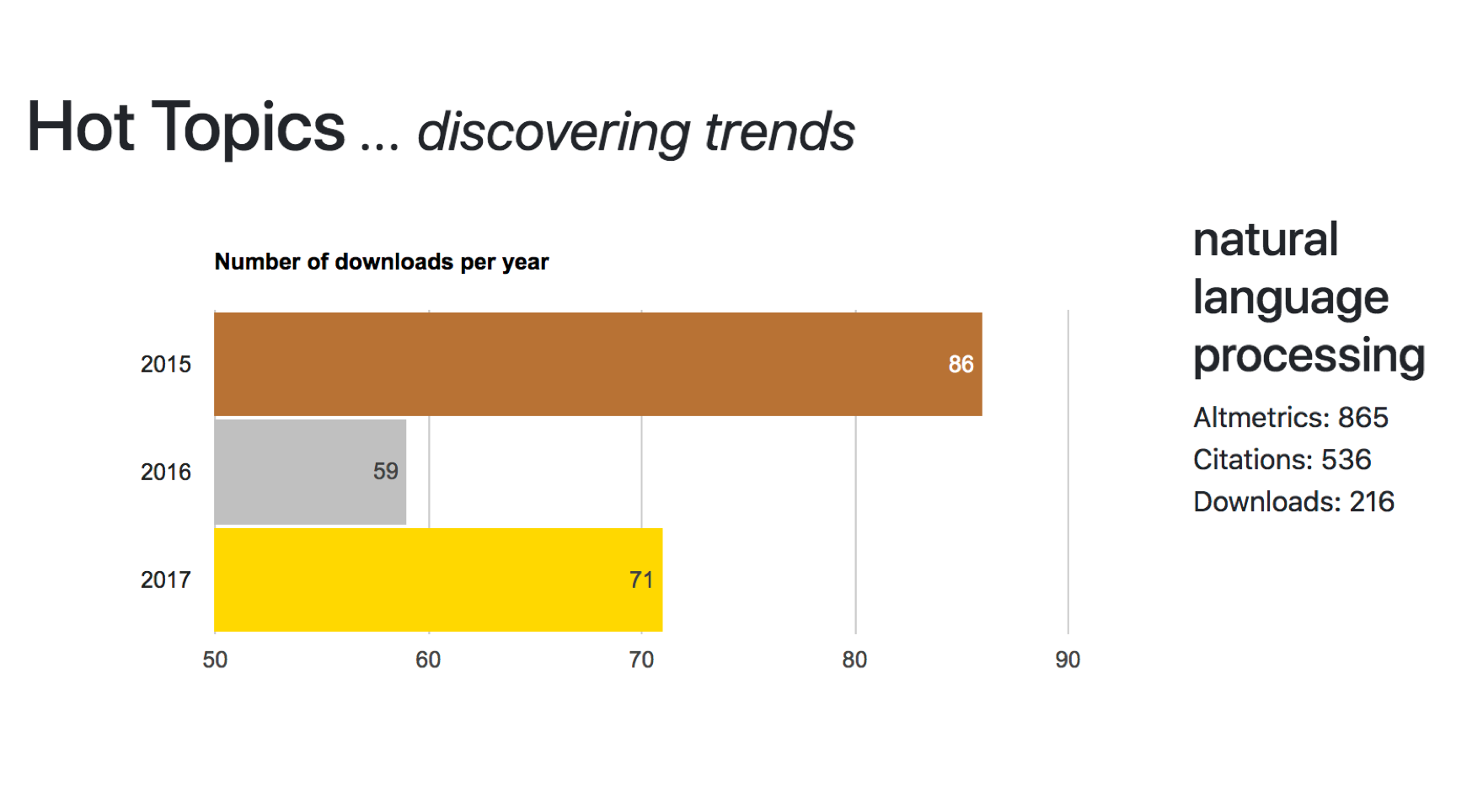
Springer Nature Hack Day – Berlin
On 26-27 April 2018, Francesco Osborne and I attended the third edition of the Springer Nature Hack Day, which was held in its headquarter in Berlin. The Springer Nature Hack Day is an event that allows researchers, developers, tech companies, and Springer Nature itself, to gather together and tackle current research issues. Offering also opportunities […]

SpringerNature Hackday – London
On the 29th November 2017, myself with two KMi colleagues (Andrea Mannocci and Thiviyan Thanapalasingam) attended the second edition of SpringerNature HackDay in London (@ SpringerNature Campus). Aliaksandr Birukou, Executive Editor of Computer Science at Springer Nature and collaborator of our research team at the Knowledge Media Institute, also joined our group on the HackDay. The whole […]
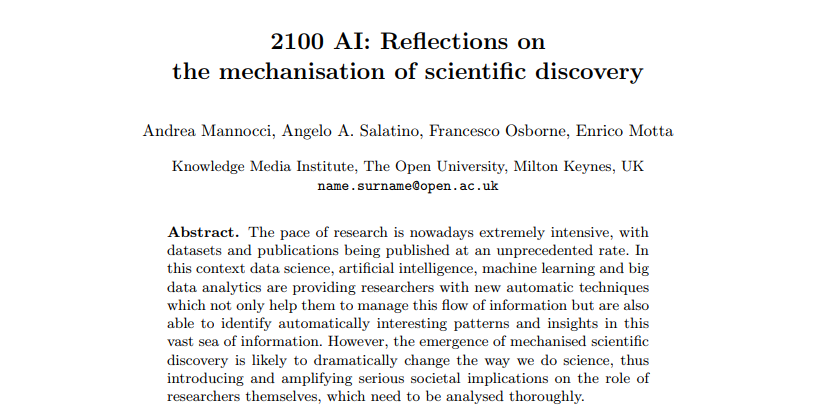
2100 AI: Reflections on the mechanisation of scientific discovery
“2100 AI: Reflections on the mechanisation of scientific discovery” is a paper submitted to the RE-CODING BLACK MIRROR Workshop co-located with the International Semantic Web Conference (ISWC) 2017, 21-25 October 2017, Vienna, Austria. Authors Andrea Mannocci, Angelo Salatino, Francesco Osborne and Enrico Motta Abstract The pace of nowadays research is hectic. Datasets and papers are […]

Smart Topic Miner
Smart Topic Miner (STM) is a web application which uses Semantic Web technologies to classify scholarly publications on the basis of Computer Science Ontology (CSO), a very large automatically generated ontology of research areas. STM was developed to support the Springer Nature Computer Science editorial team in classifying proceedings in the LNCS family. It […]

Advances Towards Early Detection of Research Topics
Acknowledging new trends in the research environment is important for many stakeholders, such as researchers, institutional funding bodies, academic publishers, and companies. In particular, being able to identify them as soon as possible can bring an important strategical advantage. A trend is usually defined as the general direction in which something is evolving. It is […]